In thinking about Net Promoter Score (NPS), I usually focus on two things:
- Its distinctive formula: NPS = Promoters – Detractors, and
- The originators’ claim that the Recommend question is the key, indeed only, question needed to assess client loyalty and satisfaction.
These raise three questions for me:
- Does the NPS formula actually add any value over, for example, just using a mean score?
- Is the Recommend question necessarily the single best question for assessing client loyalty?
- Is using a single question/metric enough to tell the whole story?
My overall conclusions are that the formula adds only little value if any; that the Recommend question is fine; and that as a single question/metric by itself can be misleading.
Does the NPS formula add value?
Many of the basic metrics used to analyse clients apply a fairly basic formula or statistic to a single question. Often this statistic is as simple as just calculating the mean or T2B. Slightly fancier metrics like the Net Value Score (NVS) or Net Promoter Score (NPS) use a Net Score type formula, such as this:
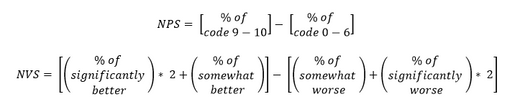
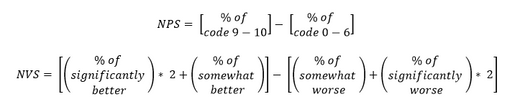
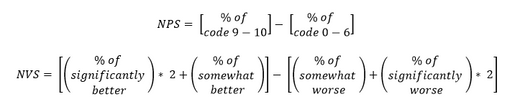
Reichheld’s arguments for the NPS formula are that it provides a simple and intuitive way to cluster clients, and understand the relative size of the clients who are showing a suite of financially advantageous behaviours, such as higher rates of repurchase and referral.
While this simple classification allows it to be used easily, it seems doubtful that such a basic classification would work consistently in every industry. This is because the amount of trust someone is taking on by following a recommendation and the amount of trust someone loses by responding to a bad recommendation differ depending on what is being recommended in the first place.
A person who recommends an accounting firm to another is putting a good deal more reputation on the line than a person who recommends a brand of soft-drink. This is because when recommending an accountant, there is more at stake for the person receiving the recommendation if it is a poor recommendation. Which in turn can have more of an impact on the relationship between the two people involved. In the soft-drink industry there is less at stake, so it easier for a person to make an off-the-cuff recommendation, but it also means that the person receiving the soft-drink recommendation will give it less attention as it is easier for someone to make the recommendation. If this is so, then in the soft-drink industry the recommendation is perhaps less of an indicator of advantageous behaviour, as the recommendation itself has less importance and commitment for both people involved. This would suggest that the classification of promoters as 9-10 is not universal in what it is measuring in different industries.
Furthermore, if the NPS formula is so simple, why can’t we just use the mean or top-2-box and get the same result? This is precisely what Hayes (2012) argues. Hayes says that in examining different statistics measures such as NPS, mean, top-box and bottom-box, these statistics measures are all highly correlated (>0.9) with each other, so that these “four metrics tell us roughly the same thing” (Hayes, 2012). This would suggest that if we were to use any of these methods to analyse the same question, they would all roughly convey the same message. In other words, no matter how we slice the question, you will still draw the same conclusions.
Research suggests that irrespective of whether you take the mean score, a net score, top- or bottom-box to analyse a metric, you will largely draw the same conclusions.
What does beaton data suggest about the formula?
Using beatonbenchmarks data, I calculated the mean score, T2B, NPS formula and a modified CSAT formula for the Recommend and Perceived Value questions.
For each of these two questions separately, correlations were run between NPS, mean score, T2B, and a modified CSAT formula to confirm if there was a strong correlation between these statistics. The correlations were based on brands that had a sample size larger than 30.
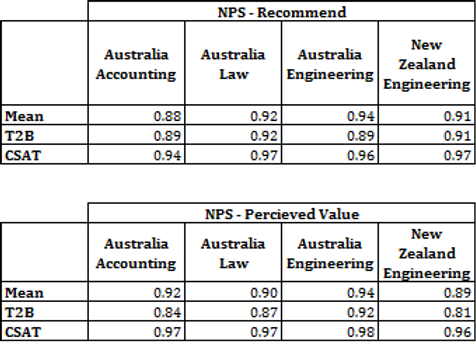
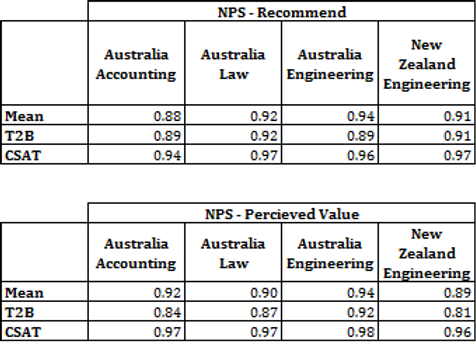
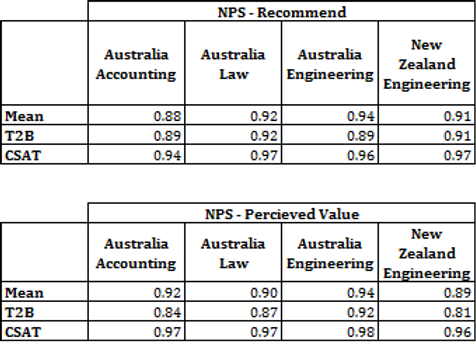
In all the tables for both questions, the correlations for NPS are all very high (>0.80). In particular, the NPS tends to be very highly correlated with the CSAT, followed by Mean and then T2B. The strength of the correlations supports Haye’s view that the different statistics will give essentially the same conclusions. If you are doing well and are amongst the better performing firms when using the mean score for Recommend, then you will still be doing well and amongst the better performing firms if you were to use the NPS formula instead.
Is the Recommend question necessarily the single best question for assessing client loyalty and satisfaction?
The debate about which question is the best at assessing client loyalty and satisfaction is certainly still open.
Reichheld, not unexpectedly, asserts Recommend is the best predictor of client loyalty, and considers Satisfaction as inferior in this task. The Recommend question does have some intuitive logic to it. If the number of respondents who are likely to recommend a firm increases, this will in turn increase actual recommendations, which in turn will lead to an increase in the number of potential clients who have positive impressions about that firm, and hopefully in turn to an increase in future sales from those potential clients. Reichheld supported the use of the Recommend question by stating that he tested 20 questions to see which one was most strongly correlated with repeat purchases or referrals. Reichheld found that the Recommend question had the strongest correlation in “most industries” (Reichheld 2003).
However, many researchers have not been able to replicate Reichheld’s link between NPS based on the Recommend question and growth. Van Doorn, Leeflang and Marleen have argued that average satisfaction is at least as good in predicting future business performance (Doorn, Leeflang, & Tijs, 2013). While Hayes argues that there is no evidence that the Recommend question is better at predicting business growth than any other question such as Satisfaction (Hayes, 2008). Furthermore, Reichheld himself says that the Recommend question only had the best correlation in “most” of the industries tested (Reichheld 2003), not all industries. But when you are promoting NPS and the Recommend question using such lines as the “ultimate question” (Reichheld 2006), and the “one number you need to know” (Reichheld 2003), Reichheld’s admission that the Recommend question is not best in all industries, is hardly reassuring with regards the strong language he uses to market the NPS approach.
Is Effort a better measure?
A construct that has gained greater traction in recent years is Effort. Dixon, Freeman, and Toman for example have argued that Effort is the best predictor of loyalty, saying that “companies create loyal customers primarily by helping them solve their problems quickly and easily” (Dixon, Freeman, & Toman, 2010). They go on to say that “many managers often assume that the more satisfied customers are, the more loyal they are…we find little relationship between satisfaction and loyalty” (Dixon, Freeman, & Toman, 2010). They argue that it is how well companies deliver on their basics, and reduce customer effort, that matters more in converting customers into loyal customers.
The growing use of the Effort question perhaps reflects a shift in how marketers are understanding the relationship between firms and clients. In today’s market, clients – particularly in professional services firms – are increasingly relying on peer-to-peer communication, and less on marketing messages they receive directly from the firms themselves. Marketers can no longer hold the notion that through a well-run direct marketing campaign alone, they can lead a potential client to choose their service or firm.
Potential clients can now just go online and learn about the pros and cons of using your firm from a cloud-based group of peers. This means that clients are able to make an informed decision about using your firm themselves, irrespective of your direct marketing and sales activities.
In this respect, the Effort question which focuses more on the actual experience of the client – as viewed by the client, is a better question in gauging how clients are communicating about your firm when engaging in peer-to-peer communication online. That is, where the Satisfaction and Recommend questions are more about what you the client think of us the firm, the Effort question is more about what was it like for you the client, and so better reflects whether the personal experiences and anecdotes posted online by your clients are being communicated in a positive or negative light. As such, it better indicates effective online referrals.
Some researchers argue that it is how well companies deliver on their basics, and reduce customer effort, that matters more in converting customers into loyal customers
Should we be focusing on only one question/metric in the first place?
The ongoing debate about which question is best, would suggest that a researcher is probably not wrong in using any particular one of these questions or metrics to predict client loyalty. However, it would also suggest that there is no panacea question either, so that a prudent researcher will probably not look at a single question or metric in isolation, but use a bundle of questions or metrics before drawing any final conclusions. Reichheld on his website also claims this in a back-handed kind of way when he says, that if “you can convince a customer to spend time answering dozens of questions, you can predict that customer’s behavior more accurately than you can with one question” (Reichheld, 2006). In other words he also seems to be saying that there is no ultimate question, and that a bundle of questions is better.
This helps us lead on to the next point, that a single question or metric can be misleading if used in isolation. To see this let’s look at the below table. Say that in 2014 a firm had 10 clients, 5 of these clients had code 9-10 for the Recommend question and so are considered ‘Promoters’, while 4 clients had code 0-6 for the Recommend question, and so they are considered ‘Detractors’. In 2014 this particular firm therefore had an NPS of 10%. But in 2015, let’s say, that 2 of those who had code 0-6 were no longer clients, so that now the firm has only 8 clients. The NPS for 2015 has now increased to 38%.
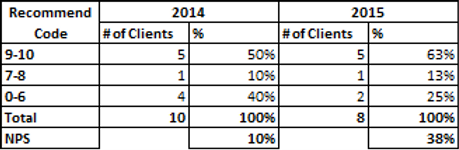
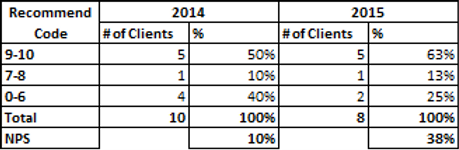
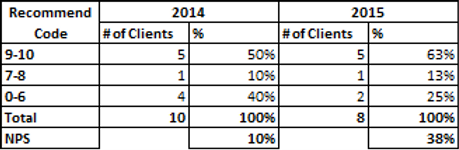
If you were to show only the NPS to your management team, they would think great, our NPS has gone up by 28 percentage points, we’re doing a fantastic job, everyone deserves a bonus. But the truth is, that while the NPS has gone up, our total number of clients has dropped. We are losing our least satisfied clients out a hole in the bottom of our bucket. This is not just an issue for the NPS either, in the above example if we had used the mean score for Recommend instead of NPS, this would also have gone up in 2015 as well. This analysis highlights it is up to the researcher to produce thorough and accurate analysis by placing the presented statistic in context – a single number by itself can be misleading.
Conclusions
This review suggests to me that if you like the NPS and find it useful, then by all means you should use it. I say this because, while the evidence suggests the NPS formula adds little additional value, and that other questions might be just as good as the Recommend question at predicting business growth, the evidence does not conclude that the NPS is incorrect or not helpful.
What I do find unhelpful though is that the NPS is sold by its creators as the ‘only’ number you need to know, a point of view that effectively encourages researchers and managers to use it in isolation and to use it as the final deciding factor.
Markets are different and firms are different, so this type of narrow NPS thinking and marketing can lead to incomplete analysis. And in turn lead people to draw incorrect or incomplete conclusions. For me, it is the marketing surrounding the NPS that is what is wrong, not the NPS itself.
The corresponding post type is disabled. Please make sure to 1) install The7 Elements plugin under The7 > Plugins and 2) enable desired post types under The7 > My The7, in the Settings section.
References
Dixon, M., Freeman, K., & Toman, N. (2010). Stop Trying to Delight Your Customers. In: Harvard Business Review, 88(7/8), 116-122.
Hayes, B. (2012, May 7). The Best Likelihood to Recommend Metric: Mean Score or Net Promoter Score? [Blog post]. Retrieved from: http://businessoverbroadway.com/the-best-likelihood-to-recommend-metric
Hayes, B. (2008). The True Test of Loyalty. In: Quality Progress, 41(6), 20-26.
Reichheld, F. (2003). The One Number You Need. In: Harvard Business Review, 81(12), 46-54.
Reichheld, F. (2006). The Ultimate Question. Driving Good Profits and True Growth. Boston: Harvard Business
Reichheld, F. (2006, July 27). Questions about NPS – and Some Answers [Blog post]. Retrieved from: http://netpromoter.typepad.com/fred_reichheld/2006/07/questions_about.html
Doorn, J.V., Leeflang, P.S.H., & Tijs, M. (2013). Satisfaction as a Predictor of Future Performance: A Replication. In: International Journal of Research in Marketing, 30(3), 314-318.